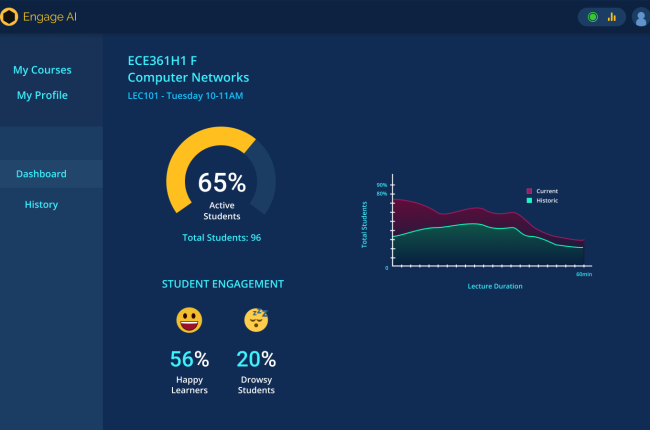
By Matthew Tierney, U of T Engineering News
Like many students around the world, Manik Chaudhery (CompE 2T0 +PEY) endured his share of virtual lectures over the past couple of years. The problem, he says, is that the format itself makes excessive demands on the instructor.
“When someone is teaching online,” says Chaudhery, “they can’t focus on the delivery and reception of the material simultaneously. There might be a hundred people or so, many with their cameras off. How do you know if your message is getting across to the students?”
The challenge of replicating a live response in an online lecture setting was a perfect one for ECE’s fourth-year Capstone course. Throughout the winter and spring of 2021, Chaudhery and his teammates Janpreet Singh Chandhok (CompE 2T0 + PEY), Raman Mangla (CompE 2T1) and Jeremy Stairs (CompE 2T0 + PEY) worked with Professor Hamid S. Timorabadi (ECE) to develop a solution.
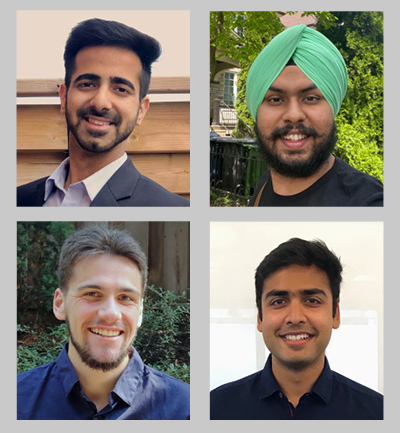
The result is Engage AI, a real-time feedback system that analyzes the facial expressions of audience members to determine whether they are ‘happy’ or ‘drowsy.’
The app displays the aggregate data in a dashboard to the lecturer, with the current ‘active’ student level — that is, those looking at the screen — displayed as a percentage.
“The app takes a 3D picture of your face and extracts the features around your eyes, nose and lips,” says Chandhok. “Then our machine learning model analyzes the expression and classifies it as an emotion.”
Machine learning (ML) helps address the problem of scale: while humans are reasonably good at judging facial expressions, we can easily be overwhelmed by a grid of many dozens of 2D faces in an online conference. The app also addresses the issue of privacy.
“Our app doesn’t require the camera to be on in Zoom or Teams or other video services, and we don’t record any of your personally identifiable information,” says Mangla. “All the image processing is done locally on your computer and our servers only receive the analyses.”
Timorabadi was impressed with the final product, which received a Certificate of Distinction and shared the Capstone Administrators’ Award for 2020. He suggested that the team submit an abstract proposal to the 2021 American Society for Engineering Education (ASEE) Virtual Annual Conference. It went on to win a Best Paper award in the team’s division.
The ML model at the core of Engage AI was developed by the students themselves. After training it with over 5,000 images, the model was able to recognize emotions with 98% accuracy and an error percentage of 4%.
The model was then confirmed with pilot runs in two online classes taught by Timorabadi. During the first test class, the team directed the students on how to act: when to close their eyes, when to smile and when to look at their phones. In the second class, they allowed the students to react naturally to an ongoing lecture.
A team member would then join the video stream after an occurrence to ask students how attentive they felt at the moment just passed. They knew they were on to something when they saw how well the responses correlated to what was being measured via the facial expressions.
“Survey results also indicated that attentiveness grew when the professor started speaking on a new topic or the slide changed. That’s what our data showed as well,” says Chaudhery.
While the original plan was to identify eight different emotions, the first pilot showed that the image processing time was creating a noticeable lag in the app’s response. Focusing on two pertinent classifications — happy or drowsy — not only made the app faster but also addressed Timorabadi’s observation that, as a lecturer, he wanted to know one thing from the app: are the students engaged or not?
“The main takeaway from the two pilot runs was: make it simple. All the bells and whistles don’t matter much,” says Stairs.
After each pilot run, the team conducted an anonymous survey after class to determine the participants’ overall attention level. Over 78% of students reported that they potentially felt more engaged, which the team attributed to in-class adjustments Timorabadi had made based on the app’s live feedback.
“Likely some version of online instruction or meetings will continue after the pandemic,” says Timorabadi. “Teachers always look for ways to reach their students — part of that is adjusting your lesson as you read the room. Engage AI mimics those valuable in-person responses. It was a timely and well-executed project.”
Professor Deepa Kundur, Chair of ECE, notes that the team’s reframing of the solution exemplifies a solid principle of engineering.
“The team learned to listen to the end-user and focus on quality over quantity,” she says. “Practical experiences like that are what make the fourth-year Capstone project such an important pedagogical component. To see our undergraduates recognized internationally is a proud moment for the department.”
After their paper presentation at the ASEE conference, a professor from the University of California, Riverside, approached the team’s representative, hoping to test the app with his own class this fall. Though everyone has gone their separate ways after graduation, to jobs across the country and globe, it might be time to get the team back together.
“Nothing’s stopping us from making this a company,” says Chaudhery.